Monitoring and Observability of messaging and middleware has and will continue to be a function of increasing importance and this is especially true for organizations in the Financial Services industry. In the financial services industry, observability refers to the ability to monitor, measure, and analyze the performance, health, and security of financial systems, applications, messaging and middleware which power long running processes in real-time.
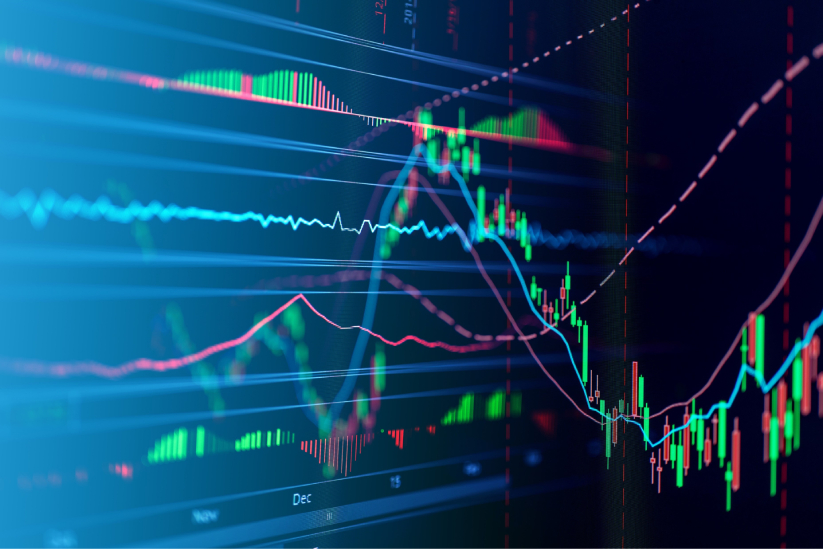