7 expert recommended data observability tools
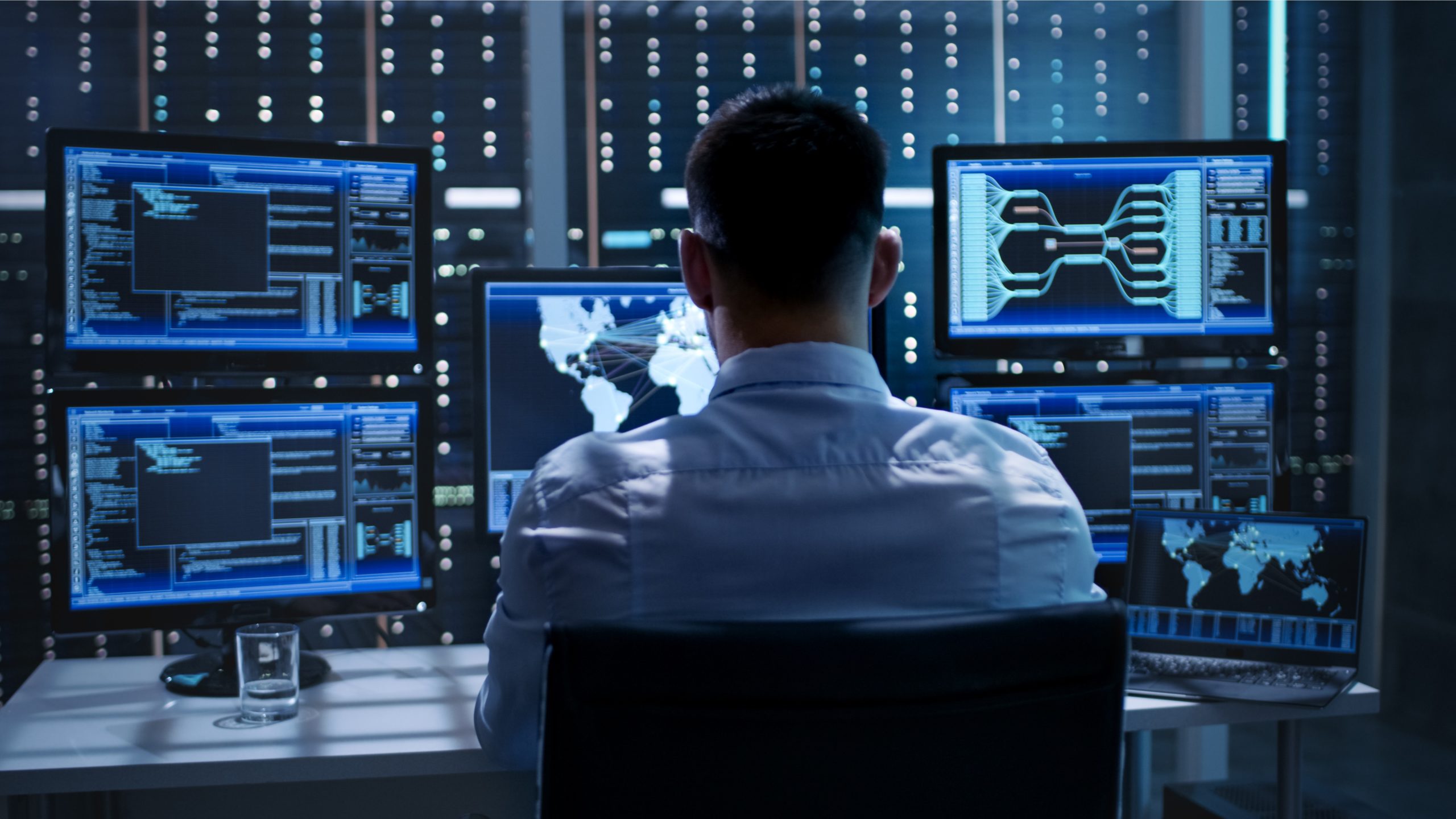
Analysts and end users have sought data observability for years, but a recent shift has changed how business processes use these tools, leaving organizations with plenty to consider when selecting which tool is best to use and if commercial investment is worth it.
Observability tools have traditionally focused on capturing and analyzing log data to improve application performance monitoring and security.
Data observability turns the focus back on the data to improve data quality, tune data infrastructure and identify problems in data engineering pipelines and processes.
“Data analysts and business users are the primary consumers of this data,” said Steven Zhang, director of engineering at Hippo Insurance. “But it’s becoming increasingly common that data engineers, who produce this data alongside product engineers, are also struggling with it.”
This calls into question the trustworthiness of the data in terms of accuracy, reliability and freshness. This is where data observability tools come into play.
A good data observability tool captures these problems and presents them in a clean structure. It helps consumers understand conceptually where the data went wrong and helps engineers identify the root causes.
There are many open source and commercial tools for organizations implementing data observability workflows. Commercial tools can fast-track this process with pre-built components for common workflows and offer plenty of vendor support. They also include better support for important enterprise use cases like data quality monitoring, security and improved decision-making. “A modern data infrastructure is often a combination of best-in-class but disjointed set of software environments that requires to be monitored and managed in a unified manner,” said Sumit Misra, vice president of data engineering LatentView Analytics, an analytics consultancy. For example, when a data job fails in an environment, another seemingly unrelated data environment must know and react to the job’s failure.
Observable, responsive and self-treating data flows are becoming essential for businesses. Commercial data observability tools can help organizations accelerate their time to deliver value from data quality initiatives, particularly when they are small or employ more business talent than IT talent, Misra said.
What to look for in a data observability tool Enterprises often end up deploying more tools than required or incorporating tools that are not specific or relevant to their business cases. "Investments in commercial data observability tools and initiatives need to be made from the perspective of the overall business, internal users and customers," said Alisha Mittal, a vice president in IT services at Everest Group. Investments in commercial data observability tools and initiatives need to be made from the perspective of the overall business, internal users and customers. More tools do not always mean higher visibility. In fact, at times, these tools increase the system's complexity. Enterprises should strategically invest in observability tools by examining their current architecture, IT operations landscape and the skill development training and hiring required to handle the tools. Various data quality and security functions are conventionally performed by the data teams of an organization. However, the value of data observability tools lies in how these activities fit into the end-to-end data operations workflow and the level of context they provide on data issues. Enterprises should consider how different data observability functions align with the following data quality management processes, Mittal said: Alerting produces alerts/notifications both for expected events and anomalies. Tracking provides the ability to set and track specific data-related events. Logging keeps a record of events in a consistent way to facilitate quicker resolution.
This article originally appeared on 7wdata.be. To read the full article, click here.